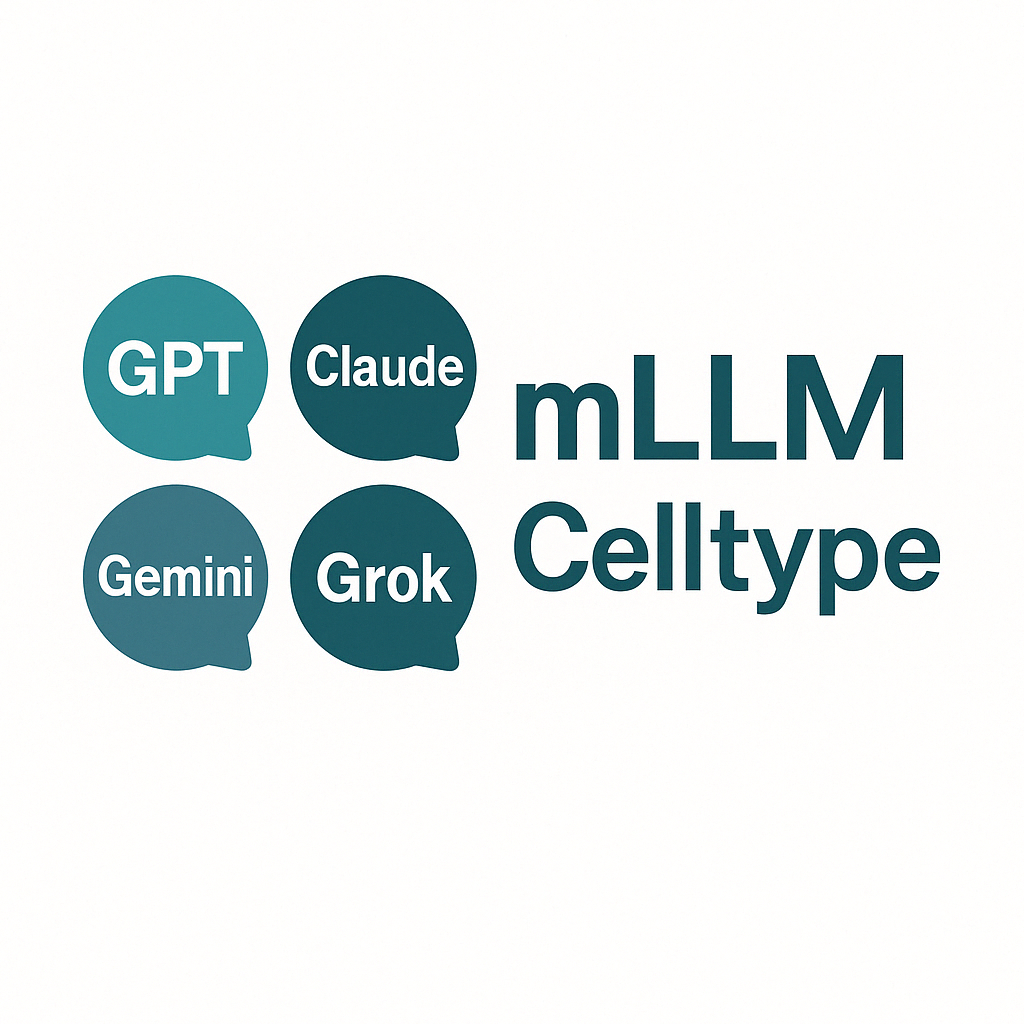
Cell Type Annotation with Multi-LLM Framework
Source:R/cell_type_annotation.R
annotate_cell_types.Rd
A comprehensive function for automated cell type annotation using multiple Large Language Models (LLMs). This function supports both Seurat's differential gene expression results and custom gene lists as input. It implements a sophisticated annotation pipeline that leverages state-of-the-art LLMs to identify cell types based on marker gene expression patterns.
Usage
annotate_cell_types(
input,
tissue_name = NULL,
model = "gpt-4o",
api_key = NA,
top_gene_count = 10,
debug = FALSE
)
Arguments
- input
One of the following:
A data frame from Seurat's FindAllMarkers() function containing differential gene expression results (must have columns: 'cluster', 'gene', and 'avg_log2FC'). The function will select the top genes based on avg_log2FC for each cluster.
A list where each element has a 'genes' field containing marker genes for a cluster. This can be in one of these formats:
Named with cluster IDs: list("0" = list(genes = c(...)), "1" = list(genes = c(...)))
Named with cell type names: list(t_cells = list(genes = c(...)), b_cells = list(genes = c(...)))
Unnamed list: list(list(genes = c(...)), list(genes = c(...)))
For both input types, if cluster IDs are numeric and start from 1, they will be automatically converted to 0-based indexing (e.g., cluster 1 becomes cluster 0) for consistency.
IMPORTANT NOTE ON CLUSTER IDs: The 'cluster' column must contain numeric values or values that can be converted to numeric. Non-numeric cluster IDs (e.g., "cluster_1", "T_cells", "7_0") may cause errors or unexpected behavior. Before using this function, it is recommended to:
Ensure all cluster IDs are numeric or can be cleanly converted to numeric values
If your data contains non-numeric cluster IDs, consider creating a mapping between original IDs and numeric IDs:
# Example of standardizing cluster IDs original_ids <- unique(markers$cluster) id_mapping <- data.frame( original = original_ids, numeric = seq(0, length(original_ids) - 1) ) markers$cluster <- id_mapping$numeric[match(markers$cluster, id_mapping$original)]
- tissue_name
Character string specifying the tissue type or cell source (e.g., 'human PBMC', 'mouse brain'). This helps provide context for more accurate annotations.
- model
Character string specifying the LLM model to use. Supported models:
OpenAI: 'gpt-4o', 'gpt-4o-mini', 'gpt-4.1', 'gpt-4.1-mini', 'gpt-4.1-nano', 'gpt-4-turbo', 'gpt-3.5-turbo', 'o1', 'o1-mini', 'o1-preview', 'o1-pro'
Anthropic: 'claude-sonnet-4-20250514', 'claude-opus-4-20250514', 'claude-3-7-sonnet-20250219', 'claude-3-5-sonnet-20241022', 'claude-3-5-haiku-20241022', 'claude-3-opus-20240229'
DeepSeek: 'deepseek-chat', 'deepseek-r1', 'deepseek-r1-zero', 'deepseek-reasoner'
Google: 'gemini-2.5-pro', 'gemini-2.5-flash', 'gemini-2.0-flash', 'gemini-2.0-flash-lite', 'gemini-1.5-pro-latest', 'gemini-1.5-flash-latest', 'gemini-1.5-flash-8b'
Alibaba: 'qwen-max-2025-01-25', 'qwen3-72b'
Stepfun: 'step-2-16k', 'step-2-mini', 'step-1-8k'
Zhipu: 'glm-4-plus', 'glm-3-turbo'
MiniMax: 'minimax-text-01'
X.AI: 'grok-3-latest', 'grok-3', 'grok-3-fast', 'grok-3-fast-latest', 'grok-3-mini', 'grok-3-mini-latest', 'grok-3-mini-fast', 'grok-3-mini-fast-latest'
OpenRouter: Provides access to models from multiple providers through a single API. Format: 'provider/model-name'
OpenAI models: 'openai/gpt-4o', 'openai/gpt-4o-mini', 'openai/gpt-4-turbo', 'openai/gpt-4', 'openai/gpt-3.5-turbo'
Anthropic models: 'anthropic/claude-sonnet-4', 'anthropic/claude-opus-4', 'anthropic/claude-3.7-sonnet', 'anthropic/claude-3.5-sonnet', 'anthropic/claude-3.5-haiku', 'anthropic/claude-3-opus'
Meta models: 'meta-llama/llama-3-70b-instruct', 'meta-llama/llama-3-8b-instruct', 'meta-llama/llama-2-70b-chat'
Google models: 'google/gemini-2.5-pro', 'google/gemini-2.5-flash', 'google/gemini-2.0-flash', 'google/gemini-1.5-pro-latest', 'google/gemini-1.5-flash'
Mistral models: 'mistralai/mistral-large', 'mistralai/mistral-medium', 'mistralai/mistral-small'
Other models: 'microsoft/mai-ds-r1', 'perplexity/sonar-small-chat', 'cohere/command-r', 'deepseek/deepseek-chat', 'thudm/glm-z1-32b'
- api_key
Character string containing the API key for the selected model. Each provider requires a specific API key format and authentication method:
OpenAI: "sk-..." (obtain from https://platform.openai.com/api-keys)
Anthropic: "sk-ant-..." (obtain from https://console.anthropic.com/keys)
Google: A Google API key for Gemini models (obtain from https://ai.google.dev/)
DeepSeek: API key from DeepSeek platform
Qwen: API key from Alibaba Cloud
Stepfun: API key from Stepfun AI
Zhipu: API key from Zhipu AI
MiniMax: API key from MiniMax
X.AI: API key for Grok models
OpenRouter: "sk-or-..." (obtain from https://openrouter.ai/keys) OpenRouter provides access to multiple models through a single API key
The API key can be provided directly or stored in environment variables:
# Direct API key result <- annotate_cell_types(input, tissue_name, model="gpt-4o", api_key="sk-...") # Using environment variables Sys.setenv(OPENAI_API_KEY="sk-...") Sys.setenv(ANTHROPIC_API_KEY="sk-ant-...") Sys.setenv(OPENROUTER_API_KEY="sk-or-...") # Then use with environment variables result <- annotate_cell_types(input, tissue_name, model="claude-3-opus", api_key=Sys.getenv("ANTHROPIC_API_KEY"))
If NA, returns the generated prompt without making an API call, which is useful for reviewing the prompt before sending it to the API.
- top_gene_count
Integer specifying the number of top marker genes to use per cluster.
- debug
Logical. If TRUE, prints additional debugging information during execution. when input is from Seurat's FindAllMarkers(). Default: 10
Value
A character vector containing:
When api_key is provided: One cell type annotation per cluster, in the order of input clusters
When api_key is NA: The generated prompt string that would be sent to the LLM
Examples
# Example 1: Using custom gene lists, returning prompt only (no API call)
annotate_cell_types(
input = list(
t_cells = list(genes = c('CD3D', 'CD3E', 'CD3G', 'CD28')),
b_cells = list(genes = c('CD19', 'CD79A', 'CD79B', 'MS4A1')),
monocytes = list(genes = c('CD14', 'CD68', 'CSF1R', 'FCGR3A'))
),
tissue_name = 'human PBMC',
model = 'gpt-4o',
api_key = NA # Returns prompt only without making API call
)
#> {"timestamp":"2025-06-27 07:14:47","session_id":"20250627_071447","level":"INFO","message":"Unified logger initialized","context":{"session_id":"20250627_071447","log_level":"INFO","log_dir":"logs"},"pid":10663}
#> {"timestamp":"2025-06-27 07:14:47","session_id":"20250627_071447","level":"INFO","message":"Processing input with model and provider","context":{"model":"gpt-4o","provider":"openai"},"pid":10663}
#> DEBUG: Formatted lines for prompt:
#> [1] "You are a cell type annotation expert. Below are marker genes for different cell clusters in human PBMC.\n\n\n\nFor each numbered cluster, provide only the cell type name in a new line, without any explanation."
# Example 2: Using with Seurat pipeline and OpenAI model
if (FALSE) { # \dontrun{
library(Seurat)
# Load example data
data("pbmc_small")
# Find marker genes
all.markers <- FindAllMarkers(
object = pbmc_small,
only.pos = TRUE,
min.pct = 0.25,
logfc.threshold = 0.25
)
# Set API key in environment variable (recommended approach)
Sys.setenv(OPENAI_API_KEY = "your-openai-api-key")
# Get cell type annotations using OpenAI model
openai_annotations <- annotate_cell_types(
input = all.markers,
tissue_name = 'human PBMC',
model = 'gpt-4o',
api_key = Sys.getenv("OPENAI_API_KEY"),
top_gene_count = 15
)
# Example 3: Using Anthropic Claude model
Sys.setenv(ANTHROPIC_API_KEY = "your-anthropic-api-key")
claude_annotations <- annotate_cell_types(
input = all.markers,
tissue_name = 'human PBMC',
model = 'claude-3-opus',
api_key = Sys.getenv("ANTHROPIC_API_KEY"),
top_gene_count = 15
)
# Example 4: Using OpenRouter to access multiple models
Sys.setenv(OPENROUTER_API_KEY = "your-openrouter-api-key")
# Access OpenAI models through OpenRouter
openrouter_gpt4_annotations <- annotate_cell_types(
input = all.markers,
tissue_name = 'human PBMC',
model = 'openai/gpt-4o', # Note the provider/model format
api_key = Sys.getenv("OPENROUTER_API_KEY"),
top_gene_count = 15
)
# Access Anthropic models through OpenRouter
openrouter_claude_annotations <- annotate_cell_types(
input = all.markers,
tissue_name = 'human PBMC',
model = 'anthropic/claude-3-opus', # Note the provider/model format
api_key = Sys.getenv("OPENROUTER_API_KEY"),
top_gene_count = 15
)
# Example 5: Using with mouse brain data
mouse_annotations <- annotate_cell_types(
input = mouse_markers, # Your mouse marker genes
tissue_name = 'mouse brain', # Specify correct tissue for context
model = 'gpt-4o',
api_key = Sys.getenv("OPENAI_API_KEY"),
top_gene_count = 20, # Use more genes for complex tissues
debug = TRUE # Enable debug output
)
} # }